Introduction
In this blog, we will be going over the below 6 main challenges of Machine Learning:
- Insufficient quantity of training data
- Non-representative training data
- Poor quality data
- Irrelevant Features
- Overfitting the training data
- Underfitting the training data
Insufficient Quantity of Training Data
To teach a toddler what a car is, all it takes is for you to point to a car and say “car” or sometimes repeating this procedure a few times. Now the child is able to recognize apples in all sorts of colors and shapes.
Machine learning is not quite there yet; it takes a lot of data for most machine learning algorithms to work. Even for very simple tasks you typically need thousands of examples, and for complex tasks such as image or speech recognition, you may need millions of examples.
As shown in a famous paper “Scaling to very very large corpora for natural language disambiguation” that even fairly simple machine learning algorithms performed almost identically well on a complex problem of natural language disambiguation, once they were given enough data (as you can see in below Figure 1).
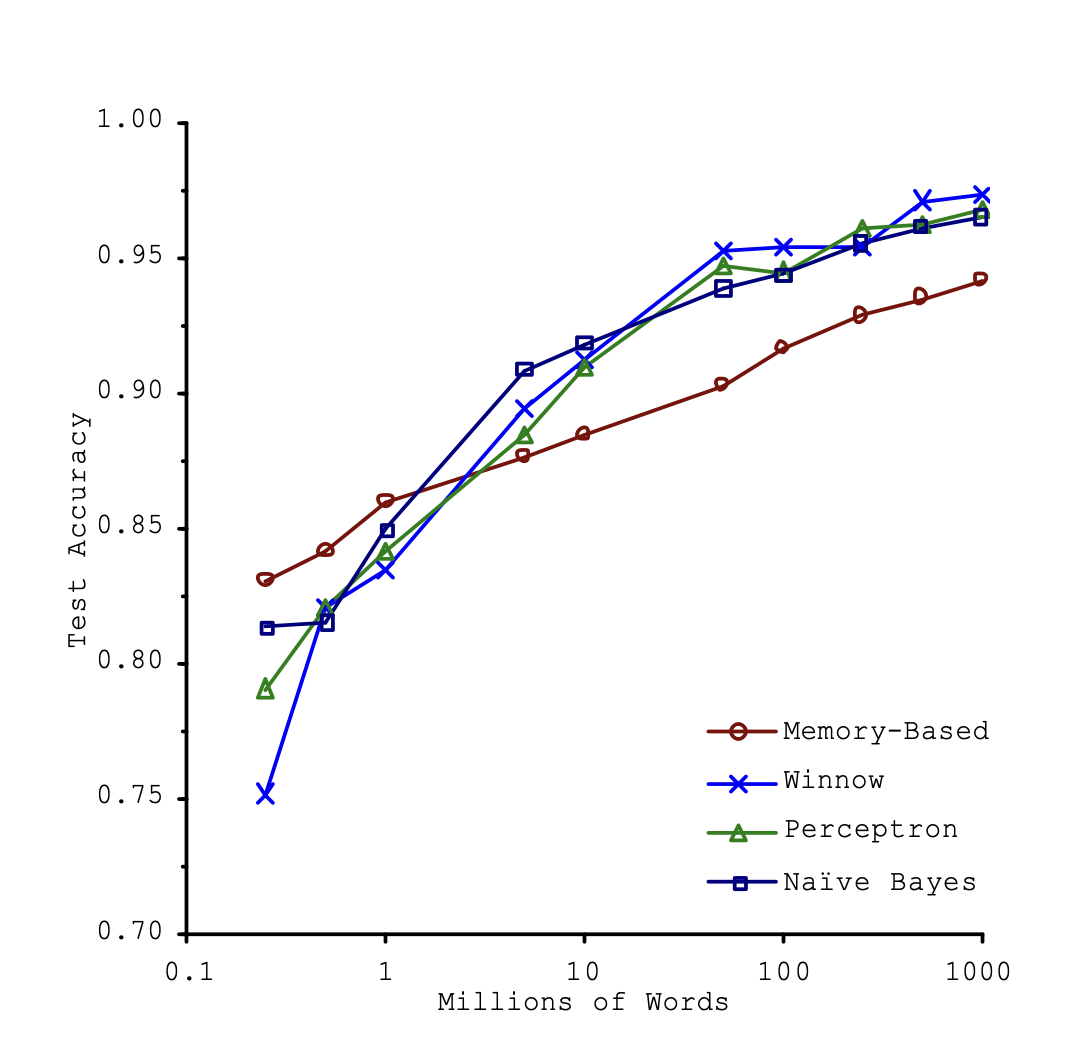
As the author put it, “these results suggest that we may want to reconsider the trade-off between spending time and money on algorithm development versus spending it on corpus development”.
Nonrepresentative Training Data
In order to generalize well, it is crucial that your training data be representative of the new cases you want to generalize to. This is true whether you use instance-based learning or model-based learning.
Having training set that is representative of the cases you want to generalize to is often harder than it sounds: if the sample if too small, you will have sampling noise, but even very large samples can be nonrepresentative if the sampling method is flawed. This is called sampling bias.
Poor-Quality Data
If your training data is full of errors, outliers, and moise, it will make it harder for the system to detect the underlying patterns, so your system is less likely to perform well. It is often well worth the effort to spend time cleaning up your training data. The truth is, most data scientists spend a significant part of their time doing just that.
The following are a couple examples of when you’d want to clean up training data:
- If some instances are clearly outliers, it may help to simply discard them or try to fix the errors manually.
- If some instances are missing a few features, you must decide whether you want to ignore this attribute altogether, ignore these instances, fill in missing values, or training one model with the features and one without it.
Irrelevant Features
Your system will only be capable of learning if training data contains enough relevant features and not too many irrelevant ones. A critical part of the success of a machine learning project is coming up with a good set of features to train on.
This process, called feature engineering, involves the following steps:
- Feature selection (selecting the most useful features to train on amoing existing features)
- Feature extraction (combining existing features to produce a more useful one)
- Creating new features by gathering new data
Overfitting the Training Data
Overgeneralizing is something that we humans do all that often, and unfortunately machines can fall into the same trap if we are not careful. In machine learning, this is called overfitting: it means that the model performs well on the training data, but it does not generalize well.
Complex models such as deep neural networks can detect subtle pattterns in the data, but if the training set is noisy, or if ie is too samll, which introduces, sampling noise, then the model is likely to detect patterns in the noise itself.
Overfitting happens when the model is too complex relative to the amount and noisiness of the training data. Here are possible solutions:
- Simplify the model by selecting one with fewer parameters, by reducing the number of attributes in the training data, or by constraining the model
- Gather mode training data
- Reduce the noise in the training data
Underfitting the Training Data
Underfitting occurs when your model is too simple to learn the underlying structure of the data. For example, a linear model is used to train on image classification; data is just more comples than the model, so its predictions are bound to be inaccurate, even on training examples.
Below are the options for fixing this problem:
- Select a more powerful model, with more parameters
- Feed better features to the learning algorithms
- Reduce the constraints on the model
References
- Géron, A. (2022). Hands-On Machine Learning with Scikit-Learn, Keras, and TensorFlow: Concepts, Tools, and Techniques to Build Intelligent Systems. Japan: O’Reilly Media.